2024年12月26日,DeepSeek-V3横空出世,以其卓越性能备受瞩目。该模型发布即支持昇腾,用户可在昇腾硬件和MindIE推理引擎上实现高效推理,但在实际操作中,部署流程与常见问题困扰着不少开发者。
本文将为你详细阐述昇腾DeepSeek模型部署的优秀实践。
昇腾DeepSeek模型部署的常见问题及解决方案见:DeepSeek在昇腾上的模型部署 - 常见问题及解决方案https://modelers.cn/updates/zh/modelers/20250226-deepseek-bestpractice-acend-deploy-part2/
01 硬件要求及组网推荐参考配置如下,部署DeepSeek-V3/R1量化模型至少需要多节点Atlas 800I A2(8*64G)服务器。
本方案以DeepSeek-R1为主进行介绍,DeepSeek-V3与R1的模型结构和参数量一致,部署方式与R1相同。
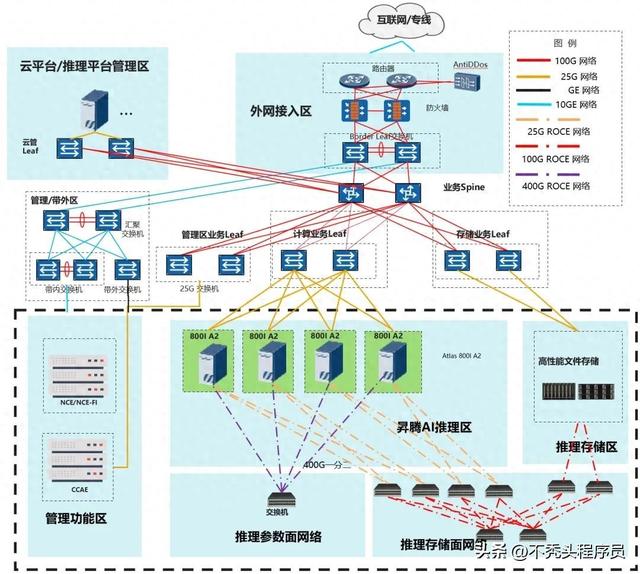
推荐使用镜像部署
1、镜像部署
昇腾官方在Ascend hub提供环境示例镜像,含推理部署配套软件以及模型运行脚本,用户可参考构建运行环境镜像进行部署。
镜像部署及启动参照ModelZoo指南中“加载镜像”章节,该指南中还包含“容器启动”等指引: https://gitee.com/ascend/ModelZoo-PyTorch/tree/master/MindIE/LLM/DeepSeek/DeepSeek-R1#加载镜像
镜像申请/下载(含于上述指南):https://www.hiascend.com/developer/ascendhub/detail/af85b724a7e5469ebd7ea13c3439d48f

2、裸机部署
根据MindIE安装指南安装软件包和运行依赖软件。
安装指南:
根据指南安装全部软件包和环境https://www.hiascend.com/document/detail/zh/mindie/100/envdeployment/instg/mindie_instg_0001.html
模型获取:https://modelers.cn/models/MindIE/DeepSeek-R1
03 权重文件准备BF16权重下载:https://modelers.cn/models/State_Cloud/DeepSeek-V3-BF16
INT8量化后权重下载:https://modelers.cn/models/State_Cloud/DeepSeek-R1-W8A8/tree/main
如已下载BF16模型,也可采用以下步骤进行模型量化,权重BF16->INT8转换预计7~8小时。
Step1:安装ModelSlimgit clone https://gitee.com/ascend/msit.gitcd msit/msmodelslimbash install.shStep2: 运行量化命令cd msit/msmodelslim/example/DeepSeek/python3 quant_deepseek_w8a8.py \ --model_path {浮点权重路径} \ --save_path {W8A8量化权重路径}更多详细量化教程请参考 DeepSeek量化文档 https://gitee.com/ascend/msit/tree/br_noncom_MindStudio_8.0.0_POC_20251231/msmodelslim/example/DeepSeek
Msmodelslim 代码仓 https://gitee.com/ascend/msit/tree/br_noncom_MindStudio_8.0.0_POC_20251231/msmodelslim
04 运行前检查服务器检查:https://gitee.com/ascend/ModelZoo-PyTorch/tree/master/MindIE/LLM/DeepSeek/DeepSeek-R1#前置准备
软件版本配套检查,含:HDK、CANN、PTA、MindIE、MindStudio
1、检查组网链接状态
a) 检查物理链接
for i in {0..7}; do hccn_tool -i $i -lldp -g | grep Ifname; doneb) 检查链接情况
for i in {0..7}; do hccn_tool -i $i -link -g ; donec) 检查网络健康情况
for i in {0..7}; do hccn_tool -i $i -net_health -g ; doned) 查看侦测ip的配置是否正确
for i in {0..7}; do hccn_tool -i $i -netdetect -g ; donee) 查看网关是否配置正确
for i in {0..7}; do hccn_tool -i $i -gateway -g ; donef) 检查NPU底层tls校验行为一致性,建议全0
for i in {0..7}; do hccn_tool -i $i -tls -g ; done | grep switchg) # NPU底层tls校验行为置0操作
for i in {0..7};do hccn_tool -i $i -tls -s enable 0;done2、根据组网设置准备rank_table_file.json
使用多节点推理时,需要将包含设备ip,服务器ip等信息的json文件地址传递给底层通信算子。参考如下格式,配置rank_table_file.json:
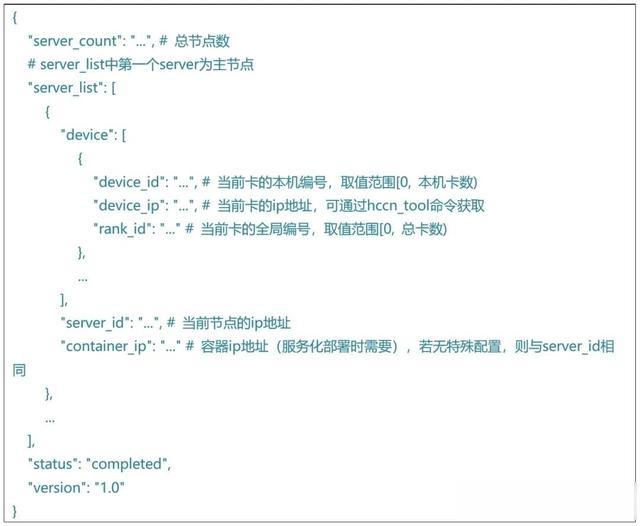
独立模型:https://modelers.cn/models/MindIE/DeepSeek-R1
服务化部署:
1、运行指南 https://modelers.cn/models/MindIE/DeepSeek-R1/blob/main/README.md(服务化测试)
2、服务启动 https://www.hiascend.com/document/detail/zh/mindie/100/mindieservice/servicedev/mindie_service0004.html
3、接口指引 https://www.hiascend.com/document/detail/zh/mindie/100/mindieservice/servicedev/mindie_service0062.html
06 模型运行1、纯模型测试
模型脚本已预制在镜像中,参照以下链接即可拉起精度测试及模型测试https://modelers.cn/models/MindIE/DeepSeek-R1/blob/main/README.md (纯模型测试部分)
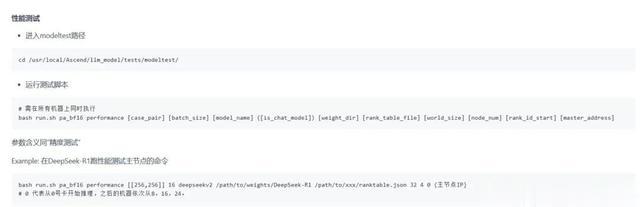
2、服务化测试
1. 运行指南 https://gitee.com/ascend/ModelZoo-PyTorch/tree/master/MindIE/LLM/DeepSeek/DeepSeek-R1#服务化测试
2. 服务启动 https://www.hiascend.com/document/detail/zh/mindie/100/mindieservice/servicedev/mindie_service0004.html
3. 常用接口指引 https://www.hiascend.com/document/detail/zh/mindie/100/mindieservice/servicedev/mindie_service0062.html